Rhetorical DIKW: Pedagogical Metalanguage for AI and Beyond
Patrick Love Monmouth University
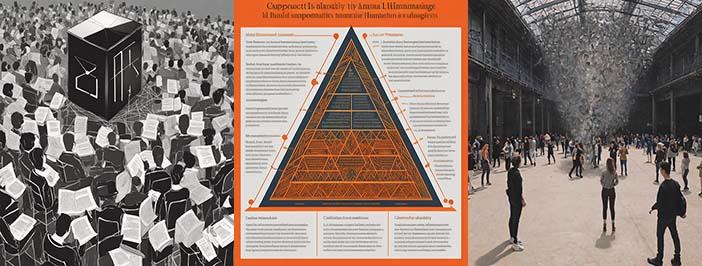
Introduction
Crypto currency and blockchain architecture, having suffered major public downturns, receded in late 2022 as if making way for mainstream generative artificial intelligence (GenAI) products, replacing decentralized ledgers in public imagination with automatically generated pictures and text via OpenAI’s DALL-E and ChatGPT. An emblematic response was Stephen Marche’s December 2022 article declaring ChatGPT and its underpinning product, GPT-3, the death of the “college essay,” heralding yet another crisis in the humanities, and questioning the value of writing and the humanities in education. Rhetoric and Composition scholars will note the tropes in Marche’s prose implying that writing primarily serves transactional purposes, particularly in academia. Marche’s declaration that the “undergraduate essay” is how “we teach (students) to research, think, and write” is easy enough to get behind, but the point is muddied by a subsequent claim. An unnamed Toronto Associate Professor (presumably of Business) claims that, when writing assignments ask “specific questions that involve combining knowledge across domains,” ChatGPT “is frankly better than the average MBA at this point” (Marche 2022). Marche’s implied concern is that AI writing can now recreate Knowledge, provided Knowledge is a compelling summary, comparison, or analysis of other writing.
One might understand pedagogical writing and humanities disciplines (like Marche implies) as existing to produce compelling understandings of the present via evidence from the past, placing the future outside its domain (like ChatGPT announcing its data cutoff on startup). This claim is both enabled and undermined by Information Theory, the mathematical basis for constituting knowledge in big-data empiricism: Information Theory translates knowledge-work between human and machine but also requires rhetorical labor and human sociality. This article will examine this tension: Information Theory’s dominant role in empiricism, particularly in digital spheres, and how it incorporates fundamental humanist concerns that an AI, working alone, cannot account for. Furthermore, this chapter will present an Information Theory-inflected approach to composition pedagogy, referred to as Rhetorical DIKW, that treats Information Theory as a boundary object bridging humanist and STEM concerns when teaching about AI, teaching AI practices, or teaching about technology and knowledge-work itself. This article will present Rhetorical DIKW as a future-oriented and intersectional approach to DIKW and its associated pyramid model, reclaiming DIKW-driven knowledge work as a rhetorical process for making and arguing for decisions based on emphasizing outcomes and their consequences. Rhetorical DIKW means taking a consciously future-oriented approach to making knowledge and ‘doing’ wisdom to make our future sustainable, just, and pleasant to inhabit by prioritizing the greatest needs in our society and ecology.
Time, Writing, and Pedagogy
Implicit in Marche’s essay on how ChatGPT fundamentally disrupts college writing is that writing to learn is primary past- and present-oriented, in that it serves to help teachers see how student think about or understand established concepts. In his 1985 article "The Language of Exclusion," Mike Rose explores what he terms the "myth of transience." This myth suggests that either the past was better than the present or that the future inevitably will be persuading us to ignore ongoing issues or adapt to them with temporary measures. This perspective aligns with a conservative mindset, assuming that challenging fundamental societal principles is unnecessary (Rose 1985, p. 600).
The myth widens the gap between the present and the future while idealizing the past. Rose argues this perspective contributes to the perpetual crises in education and writing instruction by preventing educators from seeing new ways to integrate writing into their classes and research because the future is stochastic; neither random nor predictable (Rose 1985, p. 602). The effect traps society in focus on ‘the present’ while materially continuing into the future. This disconnect echoes Paulo Freire's critique of banking education in Pedagogy of the Oppressed (1970). Banking education teaches students to accept the world as it is rather than understanding it as an ongoing process in which they actively participate (pp. 75–76).
Both Rose and Freire highlight how traditional education often emphasizes knowledge and information at the expense of action and engagement, echoing Socratic belief in dispassionate pursuit of knowledge formalized during the Enlightenment with empiricism and the scientific method (Cooper 1997, Locke 1689, Bacon 1605). To break free from this passive approach and encourage active participation in the world, Freire suggests "problem-posing" pedagogy, where students collaborate on “cognition, not transferals of information” (1970 p. 79)
To fully grasp the distinction between thought/knowledge and transferring information, especially in our digital age where transferring information is a big part of digital rhetoric, it's crucial to delve into the legacy of Information Theory and how it defines information, data, knowledge, and wisdom. This exploration can provide new insights into discussions about information and the purpose of knowledge, particularly in a world where machines, such as GenAI, play a significant role in data processing and circulation. First, we must consider how active learning pedagogy bridges Freire and Rose’s critiques and information theory’s influence.